1. Inflation Dynamics: Ornstein-Uhlenbeck Process
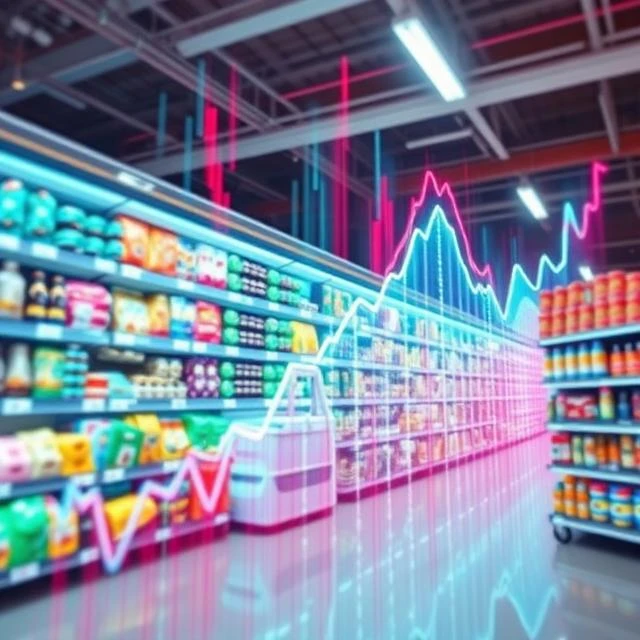
Continuous Model Justification:
This stochastic model (dπ(t) = θ(μ - π(t))dt + σdW(t)) captures inflation’s mean-reverting nature, where π(t) fluctuates around a long-term average (μ) at speed θ, while σdW(t) injects market volatility through Wiener-process shocks 💥.
By blending predictable drift (θ(μ - π(t))) with random turbulence (σdW(t)), it mirrors real-world inflation’s push-pull between stability and chaos 🎯.
The continuous framework elegantly balances persistent price trends with sudden, unpredictable jolts—perfect for simulating central bank targets amid real economic noise!